Do Perspectives on Collective Intelligence Vary Across Disciplines?
Kameda:
My background is in social psychology. I started by studying the processes through which groups form consensus through interaction, like those depicted in the movie 12 Angry Men. More recently, however, I’ve become interested in interactions that do not necessarily aim for consensus. For example, trends or fads often emerge not because someone deliberately tries to create them, but as a result of individuals acting freely. Such phenomena can be understood as macro-level patterns arising from interactions among diverse individuals. I’m particularly interested in understanding the processes through which societal consensus or common behavioral patterns emerge, even when no one is explicitly trying to achieve them. Specifically, I’d like to explore the tipping points that lead to desirable or undesirable outcomes in such processes. So when I think about collective intelligence, I broadly conceptualize it as group phenomena that produce beneficial outcomes unattainable by individuals acting alone.
Ogushi:
My foundation lies in statistical physics. During my student years, I researched nonequilibrium heat transport phenomena, exploring fundamental questions about the mechanisms behind them such as “what is heat?” and “how does heat flow cause state changes?” My main interest lies in how many-body interactions give rise to entirely different states or properties, similar to heat. Expanding this interest naturally led me to focus on the most diverse and complex system of interactions: human groups. Recently, I’ve been studying interactions within communities like Wikipedia, where individuals can freely participate, as well as the structural dynamics between articles and their editors. For me, collective intelligence encompasses not just the information itself but also the systems that create and sustain it.
Toyokawa:
My academic background is in fisheries science, rooted in an interest in ecology. In evolutionary ecology, which seeks to understand the relationship between evolutionary mechanisms and ecological dynamics, explanations often stem from a genetic evolutionary perspective. However, this framework can be extended to view individual organisms as decision-making agents. During my graduate studies under Kameda-sensei, I became fascinated by the nonlinear relationships between individuals and groups they belong to—mechanisms that cannot simply be reduced to individual decision-making processes. I first encountered the concept of collective intelligence during my undergraduate years when I studied biology, but I’ve noticed, especially in social science contexts, that the term is often discussed with varying nuances.
Kameda:
I agree—it’s difficult to pinpoint a single definition of collective intelligence. However, the key seems to lie in “interaction.” The idea is that the interactions among individuals at the micro-level can lead to macro-level states that transcend the properties of individual members. These states often have the potential to lead to relatively desirable outcomes, and I think this shared interest connects our perspectives.
Are Emergence or Self-Organization Keywords for Collective Intelligence?
Toyokawa:
In the social sciences, collective intelligence often carries the sense of using statistical aggregation of information to minimize noise. In fields like animal behavior and collective behavior studies, on the other hand, collective intelligence refers to dynamic processes of information aggregation. For example, honeybees can collectively select the optimal site for a new nest through recursive interactions, known as “waggle dances.” This type of collective intelligence emerges solely through local interactions between neighboring individuals, solving an optimization problem at the group level without top-down information aggregation mechanisms.
Now, what interests me most is the dynamics of human societies, which seem distinct from both of these examples. Humans have culture and the ability to actively modify their environments. Thus, rather than merely seeking the optimal solution for the present state, humans can engage in “niche construction,” creating new environments that lead to entirely new optimal solutions. This suggests a different way of thinking about collective intelligence.
Kameda:
If I understand correctly, what you’re saying is that the classical notion of collective intelligence involves solving optimization problems where the objective function is fixed, whereas in human societies, the objective function itself may not be fixed. Instead, it evolves dynamically as behavior expands and reshapes the environment. This concept of emergence that you mentioned seems to be a critical keyword for collective intelligence.
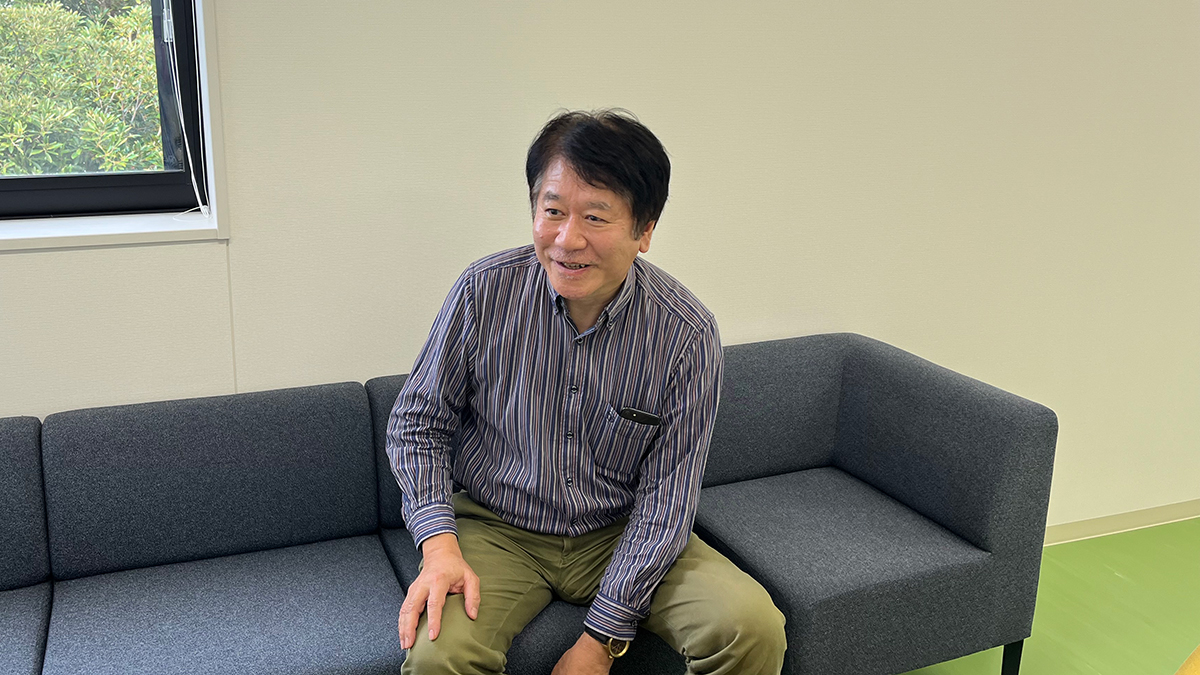
Ogushi:
In physics, we don’t often use the term “emergence” in its typical sense in Japanese, but if I had to describe it, I’d say it aligns closely with the concept of self-organization.
Kameda:
Emergence and self-organization seem to overlap significantly. How would you define self-organization?
Ogushi:
I’d describe it as the process where patterns or systems arise spontaneously as a result of interactions among elements. The fact that these systems organize themselves autonomously is fascinating and central to what draws attention to this phenomenon.
Kameda:
I once heard that emergence can be defined as “surprise” (laughs). Essentially, it refers to phenomena that occur at the group level that were not anticipated at the individual level. As Toyokawa-san mentioned earlier, this aligns with the concept of nonlinearity, which is precisely what emergence represents.
Ogushi:
That’s an important point. In physics, when a few balls move and two collide, their motion can be explained using Newton’s equations of motion. However, when we consider something like water in a glass, even if we treat each water molecule as a classical ball like a glass bead following simple rules, the entire system exhibits new collective properties—the properties of “water.” The gap caused by differences in scale, and whether or not such a gap exists, is a critical point to consider.
Toyokawa:
Emergent properties—characteristics that arise from the interactions of multiple elements—are incredibly helpful for scientists. For instance, when studying water, we don’t need to go back to quantum mechanics to understand its behavior (laughs).
Ogushi:
While there’s a gap between the motion of a few water molecules and the properties of water in a glass, the properties of water in a glass and in the ocean are the same and both can be discussed as the same “water”. Identifying where such layers or gaps in properties emerge is truly intriguing. The term “collective intelligence” seems to encompass these spontaneously arising properties and non-monotonic changes in behavior.
Can Science Incorporate the Meaning of Interactions?
Toyokawa:
Social psychology textbooks often begin by declaring that social psychology is a scientific discipline that focuses on social interactions and examines group dynamics to understand the nature of these interactions.
Kameda:
I know, that idea initially sounds very inspiring, doesn’t it? Then, when you actually get into it, what you end up doing is something completely different (laughs).
Ogushi:
In the social sciences, it’s challenging just to identify and isolate the types of interactions, isn’t it?
Kameda:
Yes—for example, sociology as a discipline tends to focus on macro-level phenomena and offers various approaches. With the current trend of computational social science, there’s often a relatively dry perspective. Take, for instance, analyzing communication patterns on X (formerly Twitter). This approach typically does not deal with issues of meaning and instead explains the regularities of behavior from an external perspective, classifying it as collective behavior. However, when sociology deals with actions and group dynamics, the question of individual values or the “meaning” behind these actions inevitably comes into play. This is a domain that cannot be verified externally. Personally, I avoid such issues in my work, but could it be that the reason interactions are so complex, as Ogushi-sensei mentioned, is that human actions carry meanings that are unique to each individual?
Ogushi:
Even without the element of meaning, it’s still difficult. For instance, people don’t just communicate directly; they’re embedded in contexts like schools, workplaces, and personal interests. Communities form that encompass these individual traits. Determining what kinds of interactions exist within such diverse and complex connections is itself a challenging task. Deciding which interactions to focus on is probably one of the most interesting aspects for researchers.
Toyokawa:
For researchers, domain knowledge plays a significant role. If you’re studying humans, you consider human-specific characteristics. For fish, you might look at their field of vision, or for ants, you might examine the volatility of their pheromones. Researchers tune the details of their models according to these specific conditions. However, as the level of abstraction in mathematical models increases, you start to see similarities across domains.
Kameda:
With mathematical models, even if the subjects differ, the principles often make sense across domains, which is one of the strengths of this approach. However, when we shift to the social sciences and deal with collective action or collective intelligence, there are aspects that can’t be fully captured by mathematical models.
Toyokawa:
The issue of meaning seems similar to the question of whether we could even pose the same inquiries if we weren’t Homo sapiens. If the internal meanings and values we hold can’t be measured by other organisms, it raises the question of whether it’s even worth asking. Personally, I’d say I’m not very interested in that question (laughs).
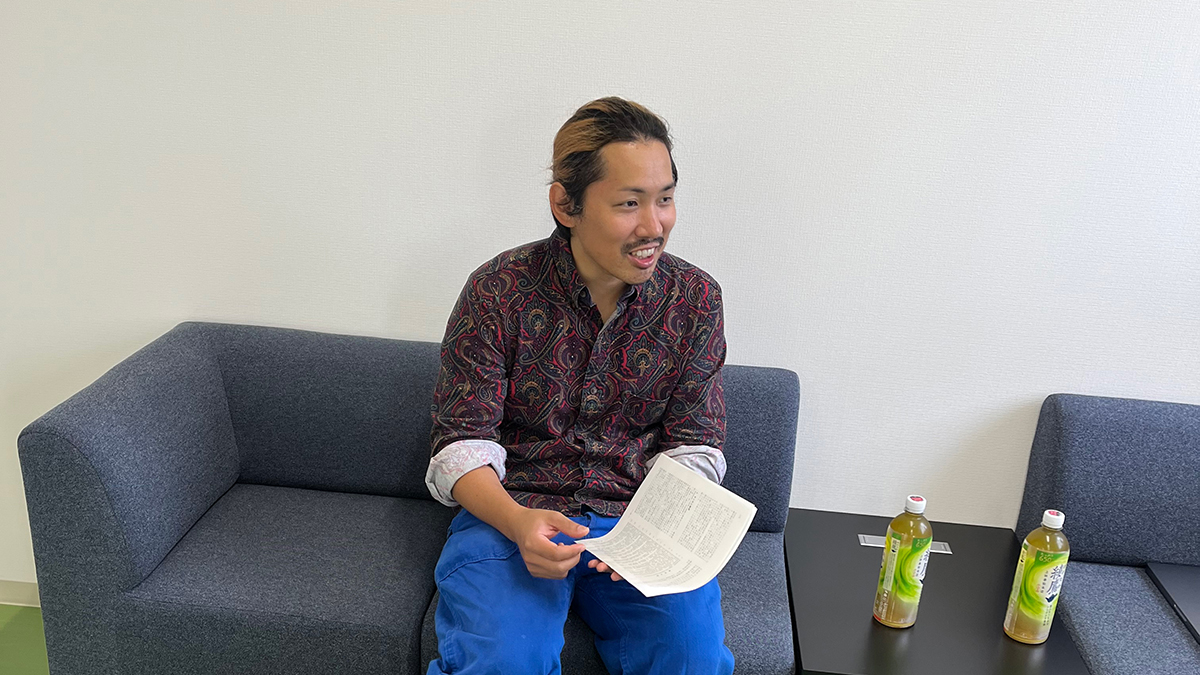
Ogushi:
It’s only through individuals’ interactions with their external environment that we can observe these phenomena.
Toyokawa:
Even if there are latent variables or processes we can’t observe, what I am interested in is behavior. So, I would treat these unseen processes as latent values and estimate them in our research.
Kameda:
From an external observational perspective, we can infer the values of individuals, but we can never truly capture their subjective experiences. I suppose that’s the nature of science.
Toyokawa:
While I can empathize with these ideas from an entertainment perspective, I feel that the scientific approaches to them still seem immature—the methodologies aren’t quite there yet.
Why Does Wikipedia Work So Well?
Kameda:
Could you briefly introduce your specific research on collective intelligence?
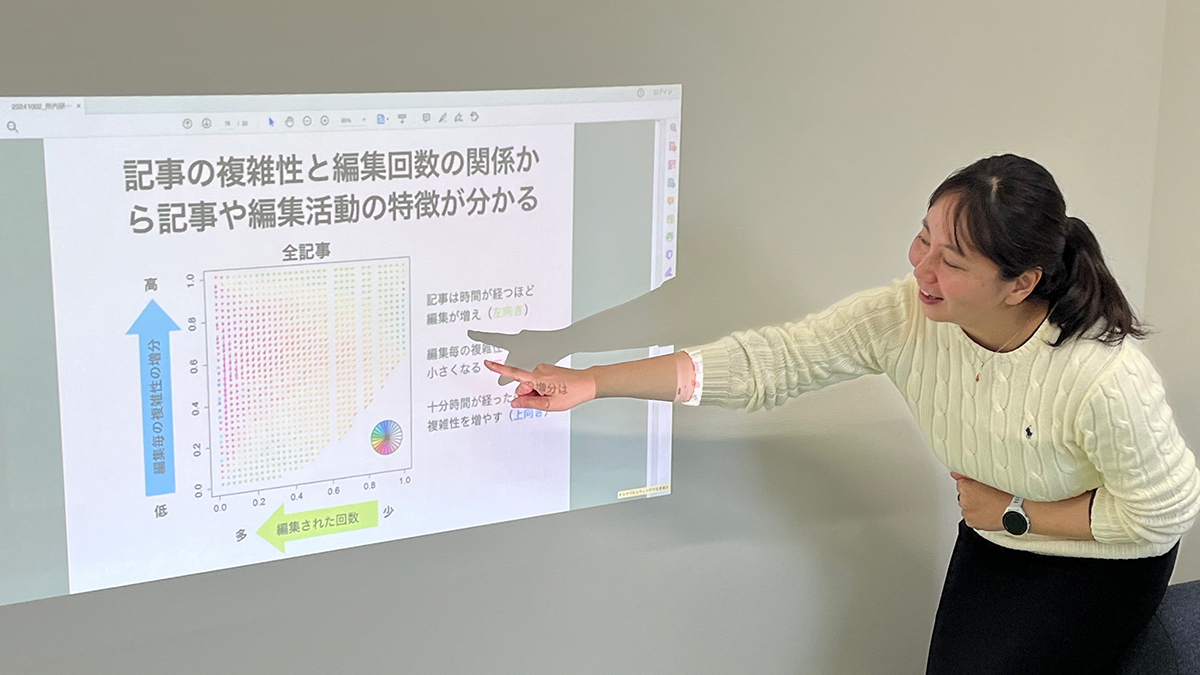
Ogushi:
Wikipedia is an online encyclopedia where anyone can freely edit or create articles. Despite this openness, it somehow functions effectively as a system. Both the articles and the editors are highly diverse in terms of their topics of interest and abilities, so simply counting the number of edits doesn’t adequately capture the complexity of the articles or the nature of the editors. Assuming that the quality and characteristics of articles and editors are consistently determined by the entire network of editing relationships, we analyzed this network. We found that Wikipedia’s editing network has a structure resembling a kind of ecosystem, with diverse articles and editors coexisting (Figure 1).
Moreover, modeling based on the results of our data analysis revealed that the collaborative editing process on Wikipedia hinges on two key tendencies among individuals: a “maintenance-like edit” and a “content edit.” Incorporating these two tendencies as intrinsic traits of editors allowed us to effectively explain one aspect of collective intelligence in this context.
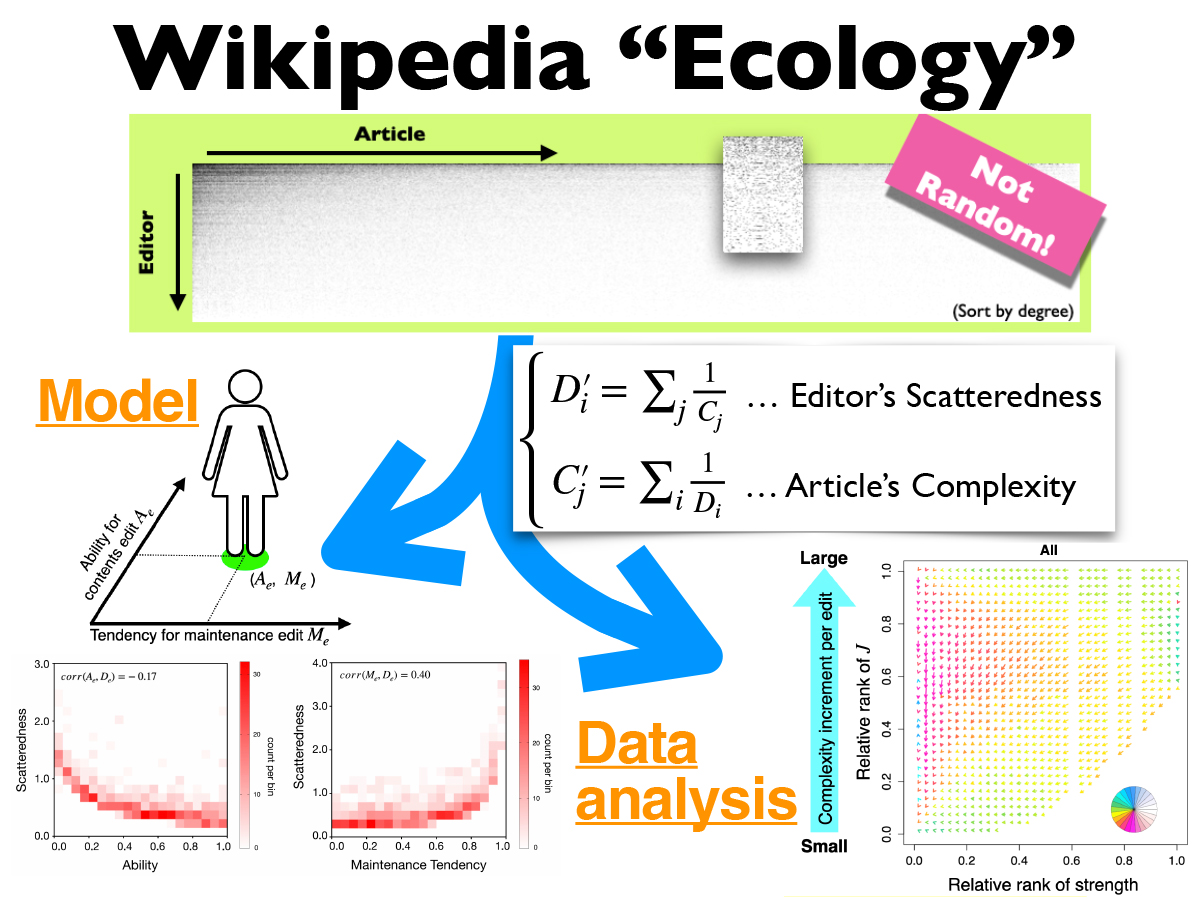
Figure 1
Learn From Others to Improve Yourself?
Toyokawa:
I’ve conducted research on the behavior of reinforcement learning agents in computer simulations concerning collective intelligence. In reinforcement learning, agents might avoid exploring certain negative choices after experiencing failure, which can hinder their ability to maximize outcomes through trial and error.
Kameda:
We can think about it using the metaphor of slot machines. Imagine spending 24 hours in Las Vegas with $2,000 to maximize your winnings across ten slot machines. Initially, you could explore all the machines with small bets, then focus on the ones that yield better results. However, if one machine has high potential rewards but also a high risk of loss, a single bad experience might lead you to avoid that machine entirely—a significant drawback.
Toyokawa:
Exactly. If you want to maximize rewards in the long run, you should ideally target the high-risk, high-reward machines. However, simple reinforcement learning often makes it difficult to achieve this. So, we considered a scenario where gamblers can observe which machines others are playing on but cannot see how much they are winning.
Kameda:
Like a long line at a popular ramen shop—you don’t know how good the food is, but you know it’s trendy (laughs).
Toyokawa:
Yes. By slightly modifying the reinforcement learning model, we introduced a parameter to represent imitation behavior. If agents completely ignore others, the value is 0; if they fully mimic others, the value is 1; and if they occasionally imitate, the value lies between 0 and 1. By adjusting this probability, we examined differences in the collective behavior of the group. Surprisingly, we found that under certain imitation probabilities, agents collectively converged on high-risk, high-reward machines, generating long-term profits.
Further analysis revealed that this emergence occurred when two mechanisms aligned: (1) agents were willing to retry machines that had previously provided a negative experience if enough others were using them, and (2) when the majority began noticing high-reward options, others would imitate this majority (Figure 2). We even conducted online experiments with human participants on slot machines and successfully replicated these patterns.
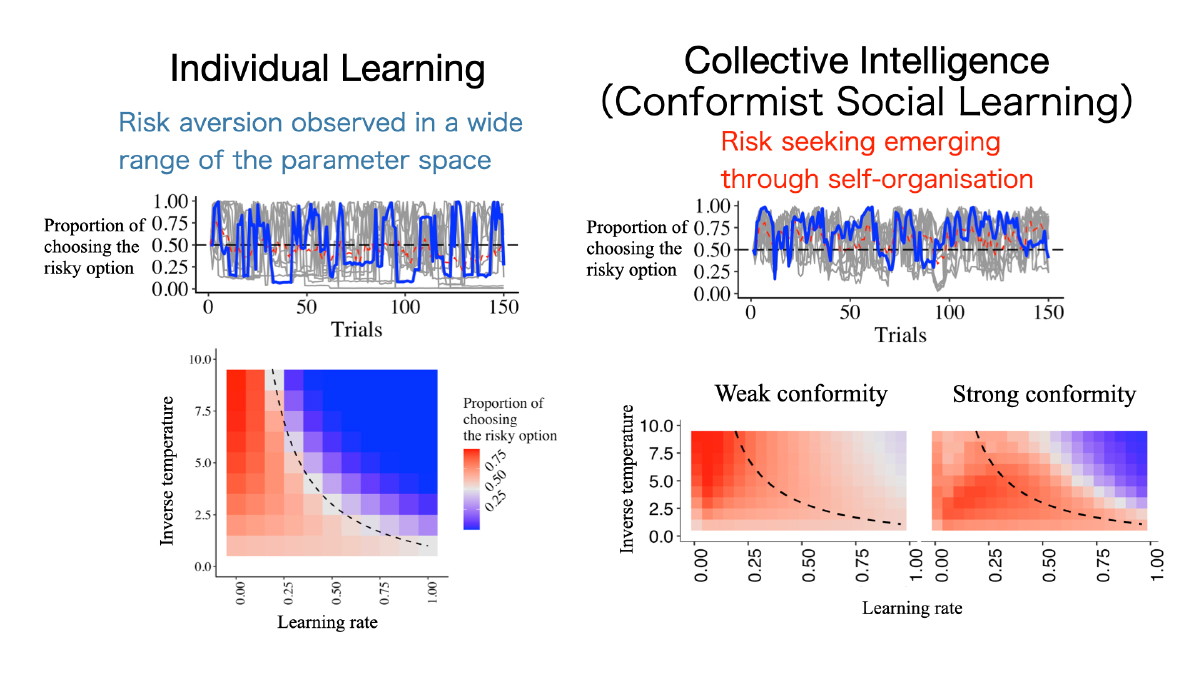
Figure 2
Ogushi:
When considering opinion formation, the concept of indirect information—relying on the overall reputation within a network rather than direct evaluations or rewards—seems crucial. In this study, did you also focus on group size or the network structure within the group?
Toyokawa:
In this research, we didn’t consider agent-to-agent network structures. Instead, we assumed everyone could see everyone else. Currently, we’re studying what happens when we vary the network structure—for instance, if agents can only see their neighbors or if they can only observe others’ actions if both parties mutually agree to exchange information.
Ogushi:
The real structure of connections seems to be a significant factor. For instance, in Wikipedia’s editing network, there are real-world properties that theoretical models cannot yet replicate. Additionally, while human societies, ant colonies, and corporate networks differ vastly in detail, network analysis reveals common properties. For example, characteristics like “small-world networks” (where average distances between nodes are surprisingly short, reflecting the “it’s a small world” phenomenon) are frequently observed in real-world networks. By focusing on network structure, we may be able to capture universal properties or features in real-world systems, if they exist, that cannot be fully understood using current models.
Cascading Behavior: Collective Wisdom from Simple Rules
Kameda:
Let me give an example of herd behavior: the phenomenon of information cascades. This occurs when individuals base their decisions on the choices of those who came before them in a sequential decision-making context. Think of situations like internet communication or stock trading, where “herd behavior” can emerge. In a simple experiment, we asked 30 participants to answer a quiz question sequentially in a chain, such as estimating the diameter of the sun. Initially, participants answered individually. Then, they were shown the average of the previous responses and had the option to revise their answers. This process continued sequentially until the 30th participant. When errors occurred early on in the chain, they could propagate through the group.
Interestingly, when we conducted this experiment in both France and Japan, collective wisdom generally emerged despite such potential pitfalls. The underlying mechanism was straightforward: individuals confident in their judgment retained their original answers, while those less confident adopted the average of others’ responses. This behavior, known as “copy when uncertain,” acts as a simple decision-making algorithm (Figure 3). If more accurate individuals are also more confident, this micro-level behavior ensures the quality of shared information in the chain and can lead to the emergence of collective intelligence as a macro-level pattern. This is a straightforward example of how basic behavioral principles can result in large-scale patterns of collective intelligence.
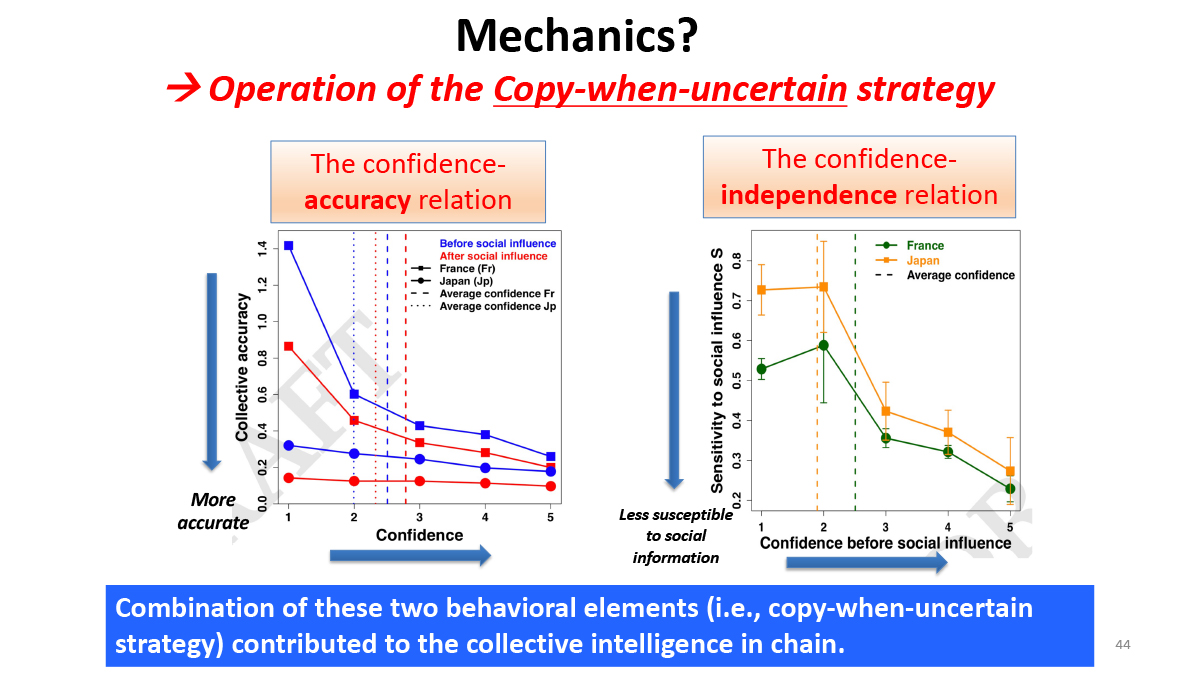
Figure 3
Embracing the Spirit of “Let’s Agree to Disagree”
Kameda:
Today, we invited an external researcher to join us, but in the future, we’re considering organizing discussions between science faculty members from the Faculty of Mathematical Informatics and humanities and social science faculty members from elsewhere in the university. Beyond these discussions, we hope this Center will serve as a foundation for various collaborations, using existing research communities to disseminate knowledge through seminars, workshops, and other initiatives.
Toyokawa:
As Ogushi-san mentioned earlier, I think the existence of mathematical models allows us to communicate with others. Even if our research topics and interests differ, having a shared language enables us to engage in constructive and creative conversations. That said, the challenge lies in addressing the “world of meaning” that Kameda-sensei mentioned earlier—how we incorporate and collaborate with that kind of knowledge might be an important task for the future.
Kameda:
I have no idea how we can overcome that challenge at the moment (laughs). But it’s definitely worth the effort!
Ogushi:
Speaking in a shared language is not easy. It’s natural for specialists in different fields to have entirely different perspectives, so repeated efforts will likely be necessary. In the world of research, disciplinary boundaries often mean little, and researchers can be united more by their methodologies than by their subject matter. Regardless of whether someone is from the humanities or the sciences, having conversations with people from seemingly unrelated fields is incredibly important for us as researchers.
Kameda:
There’s an English phrase, “Let’s agree to disagree,” which means agreeing to acknowledge our differences of opinion. I think that’s an important concept here as well. With this mindset, I look forward to fostering intellectual exchanges with researchers from a wide range of disciplines.